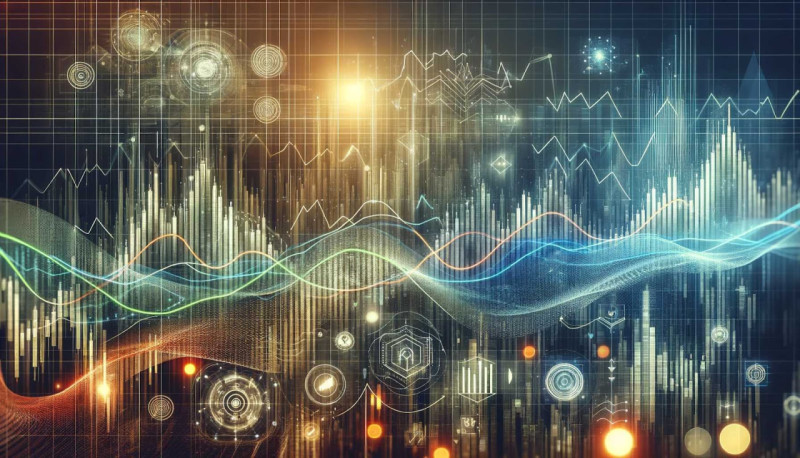
Introduction
Brief explanation of the moving average method
The moving average method stands as a cornerstone in the world of trading, revered for its simplicity and effectiveness in market analysis. This statistical technique, fundamental to technical analysis, helps traders smooth out price data to identify and validate trends in financial markets. It operates by creating an average of a security's price over a specified period, providing a dynamic yet clear view of the market's direction.
At its essence, the moving average takes a set number of past price points, adds them together, and then divides the total by the number of points. This process results in a "moving" average because with each new trading day, the oldest price in the set is replaced by the latest, ensuring the average is always current. The simplicity of this calculation belies its power: by smoothing out daily price fluctuations, it offers traders a clearer perspective on the market's true direction, free from the noise of short-term volatility.
In trading, moving averages come in various forms, each with its unique calculation and application. The most common types include the Simple Moving Average (SMA), which calculates the average price over a specific number of days, and the Exponential Moving Average (EMA), which gives more weight to recent prices in an attempt to make it more responsive to new information.
Traders rely on moving averages not just for gauging the trend direction but also for identifying potential support and resistance levels, timing entries and exits, and even predicting future price movements. When a stock price crosses its moving average, it can signal a potential change in trend, making it a critical moment for traders to assess their positions.
The moving average method's adaptability across different time frames and its compatibility with other technical indicators make it a versatile tool in a trader's arsenal. Whether you're a day trader looking at short-term price movements or a long-term investor analyzing broader market trends, the moving average can be tailored to suit diverse trading strategies.
In the following sections, we will explore the intricacies of the moving average method in trading, its different types, practical applications, and the strategic insights it offers to traders navigating the complex and often turbulent financial markets.
Importance in Various Fields
1. Finance
- Risk Management - In finance, moving averages are crucial for risk management. Financial institutions use them to understand market trends, helping to mitigate risks associated with investment portfolios.
- Portfolio Analysis - Moving averages aid in analyzing the performance of different financial assets over time, enabling portfolio managers to make informed decisions about asset allocation.
- Loan and Credit Analysis - Banks and lending institutions often employ moving averages to assess economic trends that affect interest rates, inflation, and loan default probabilities.
2. Economics
- Economic Trend Analysis - Economists use moving averages to smooth out economic data, eliminating short-term fluctuations and revealing longer-term trends in key indicators like GDP growth, unemployment rates, and inflation.
- Policy Formulation - Governments and policy-makers rely on moving averages to understand economic cycles, which is critical for formulating fiscal and monetary policies.
- International Trade Analysis - Moving averages help in analyzing trade patterns and global market trends, which is essential for international business and economic forecasting.
3. Statistics
- Data Smoothing - In statistical analysis, moving averages are used for data smoothing, reducing random "noise" and making it easier to identify meaningful trends in data sets.
- Time Series Analysis - They are extensively used in time series analysis, a statistical method vital for forecasting and predicting future values based on previously observed values.
- Quality Control - In industrial statistics, moving averages are key in quality control processes, helping to monitor and maintain product quality by detecting variations in manufacturing and production data.
4. Additional Fields
- Meteorology and Environmental Science - For forecasting weather patterns and analyzing environmental data trends.
- Public Health - In epidemiology, moving averages help track the spread of diseases and the effectiveness of public health interventions over time.
- Engineering - Used in signal processing and control systems to filter out noise and optimize system performance.
In each of these fields, the moving average method provides a simplified yet powerful way to interpret complex data sets. By smoothing data fluctuations, it helps professionals across various disciplines make more accurate predictions, understand underlying trends, and make data-driven decisions. This wide-ranging applicability underscores the method's foundational role in analytical and predictive modeling.
Basic Concept of Moving Average Method
Definition and Fundamental Principles
The moving average method is a pivotal statistical tool in financial market analysis, characterized by its ability to smooth out price data to reveal underlying trends. This method calculates an average price over a specific period, which is continually updated as new data becomes available, effectively smoothing short-term price volatility to provide a clearer long-term trend perspective.
The concept begins with the selection of an averaging period, the 'window', which can vary from days to weeks or months, tailored to the trader's strategy and objectives. Commonly used windows include 10, 20, 50, 100, and 200 days. There are several types of moving averages, each with its distinct calculation and application. The Simple Moving Average (SMA) computes the arithmetic mean of a set of values, like averaging the closing prices of the last 10 days for a 10-day SMA. On the other hand, the Exponential Moving Average (EMA) places greater weight on recent prices, making it more responsive to new information, using a more complex formula that considers all price points within the period. The Weighted Moving Average (WMA) also assigns greater importance to recent data but differs from the EMA by decreasing weights linearly rather than using exponential smoothing.
The primary goal of using a moving average is to identify and confirm market trends by filtering out the 'noise' from random short-term price fluctuations. This process involves summing up the closing prices of an asset over the chosen period and dividing this total by the number of periods, with adjustments for EMAs and WMAs due to their weighting factors.
In interpreting moving averages, traders look for several key indicators. The position of the price in relation to the moving average can signal an upward or downward trend. Additionally, moving averages can act as levels of support in a rising market or resistance in a falling market. Crossovers, where the price crosses the moving average or when two different moving averages cross each other, are particularly significant. These crossovers are often seen as signals to buy or sell and are a cornerstone of many trading strategies.
Understanding these principles is crucial for anyone looking to utilize the moving average method in trading. Its simplicity and adaptability make it a favored tool among traders, but a thorough grasp of its fundamentals is essential for effective market analysis and decision-making.
Types of Moving Averages
The moving average method in trading encompasses three primary types, each with its distinct characteristics and applications: Simple Moving Average (SMA), Weighted Moving Average (WMA), and Exponential Moving Average (EMA).
1. Simple Moving Average (SMA) - The SMA is arguably the most straightforward and commonly used type of moving average. It calculates the average price over a specific time period, usually using the closing prices. For example, a 10-day SMA would sum the closing prices of the last 10 days and divide the total by 10. The SMA treats all data points within its period equally, giving the same weighting to each price. This equal weighting approach means that the SMA may be slower to respond to recent price changes, which can sometimes delay trading signals.
2. Weighted Moving Average (WMA) - The WMA modifies the SMA approach by assigning different weights to each data point in the period. It typically gives greater weight to more recent prices, making the WMA more responsive to recent market movements. This increased sensitivity can be beneficial in fast-paced markets, where recent price action is often more reflective of the current trend. However, the heightened sensitivity also makes the WMA more vulnerable to producing false signals, particularly in volatile market conditions.
3. Exponential Moving Average (EMA) - The EMA further advances the concept of the WMA. Like the WMA, it gives more importance to recent prices, but it does so through an exponential weighting scheme, ensuring that all prices in the period have some impact on the average, albeit decreasingly for older prices. The EMA is particularly valued for its ability to reduce the lag inherent in moving averages, providing a quicker response to price changes than the SMA. This makes the EMA a preferred choice for many traders, especially those looking to capture trends early and react swiftly to market changes.
Each of these moving averages serves a unique purpose and is selected based on the trader's strategy and the specific characteristics of the market being analyzed. Understanding the differences and appropriate applications of each type is essential for effective trading and market analysis.
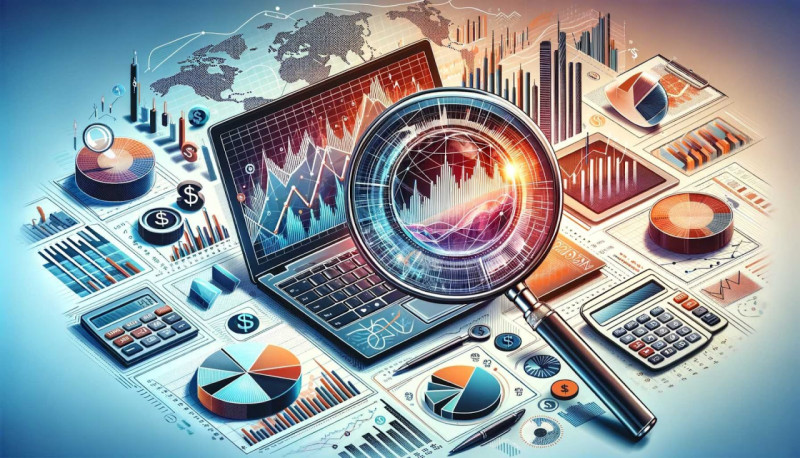
Visual Representation
Visual representation through graphs is a fundamental aspect of using moving averages in trading, as it allows traders to quickly and effectively interpret market trends and signals. Graphs with moving averages overlay the price data, enabling a visual comparison of the price movements with the moving averages. Here's how different types of moving averages are represented on graphs, with examples:
1. Simple Moving Average (SMA) Graph:
- The SMA line is plotted directly on the price chart.
- It smooths out price data by creating a constantly updated average price.
- Example: In a graph showing a 50-day SMA, the line would represent the average closing price of the past 50 days, updating daily. This line smooths out short-term fluctuations, showing the longer-term trend direction. If the price is consistently above the SMA line, it suggests an upward trend, while consistently below indicates a downward trend.
2. Weighted Moving Average (WMA) Graph:
- The WMA line, like the SMA, is plotted on the price chart but with more emphasis on recent prices.
- It reacts more quickly to price changes than the SMA due to its weighting.
- Example: On a chart, a WMA might closely follow price movements more than an SMA, quickly reflecting recent price increases or decreases. This characteristic makes it useful for traders who rely on more responsive indicators.
3. Exponential Moving Average (EMA) Graph:
- The EMA line is also plotted on the chart and gives even more weight to recent prices than the WMA.
- It is known for its quick reaction to price changes, making it ideal for identifying trends in fast-moving markets.
- Example: In a volatile market, an EMA graph would closely track price movements, swiftly adjusting to recent changes. This can be particularly evident in a short-term EMA like a 10-day EMA, where the line rapidly follows the price, showing the immediacy of its reaction to market movements.
In trading, these moving averages are often used in combination. For example, a trader might use both a short-term EMA (like 10-day) and a longer-term SMA (like 50-day) on the same graph. A crossover of these lines (where the short-term EMA crosses above the long-term SMA) can be interpreted as a bullish signal, while a crossover below may signal a bearish trend.
Graphs with moving averages offer a dynamic and intuitive way to analyze market behavior. They not only help in identifying the general trend direction but also in spotting potential reversals, support and resistance levels, and crossovers that are critical for making informed trading decisions.
Application in Financial Markets
Moving Averages in Stock Market Analysis
Moving averages play a crucial role in stock market analysis, offering traders and investors a reliable means to assess market trends and make informed decisions. Their versatility in the financial markets can be seen in several key applications.
One of the primary uses of moving averages in stock analysis is for identifying and confirming market trends. When a stock's price consistently trends above its moving average, it typically indicates an uptrend, suggesting bullish sentiment. Conversely, a stock price that remains below its moving average often signals a downtrend, indicating bearish sentiment. This trend identification helps investors and traders align their strategies with the market's direction.
Moving averages also serve as dynamic support and resistance levels in the stock market. They provide a visual guide for potential price reversals. For instance, in a bullish market, a moving average can act as a support level, hinting that the stock price might rebound if it dips but stays above the moving average. In a bearish scenario, the moving average can become a resistance level, suggesting that the stock might struggle to rise above this line.
In addition to trend analysis, moving averages are instrumental in smoothing market noise. By averaging out the prices over a specific period, they help filter out short-term volatility and random price fluctuations, offering a clearer picture of the stock's long-term trajectory. This smoothing effect is particularly beneficial in volatile markets, where it can be challenging to discern meaningful trends amid frequent price swings.
Moving averages also form the basis for various trading strategies and technical indicators. For instance, the crossover strategy, where two moving averages of different lengths (such as a 50-day and a 200-day moving average) are used in conjunction, can signal potential entry and exit points for traders. A crossover of the shorter moving average above the longer one might indicate a buying opportunity, while a crossover below could suggest a selling point.
Furthermore, moving averages are often combined with other technical analysis tools to enhance market analysis. By overlaying moving averages on price charts alongside indicators like Bollinger Bands, Relative Strength Index (RSI), or Moving Average Convergence Divergence (MACD), traders can gain a more comprehensive view of the market conditions, helping them to make more nuanced and informed trading decisions.
Overall, the application of moving averages in the financial markets underscores their value in providing a deeper understanding of market dynamics. Their ability to simplify complex price data into understandable trends makes them a staple in the toolkit of many traders and analysts, guiding them through the intricacies of market behavior.
How Traders Use Moving Averages for Trend Identification
Traders use moving averages for trend identification in the stock market, leveraging their capacity to convert complex price data into clear trends. This approach is crucial for aligning trading strategies with market movements and involves several key techniques.
A primary use of moving averages is to determine the overall direction of the market or a specific stock. If the stock price is consistently above its moving average, it is typically viewed as being in an uptrend. In contrast, a stock price that remains below its moving average is often considered to be in a downtrend. This straightforward observation allows traders to align their strategies with the prevailing market trend.
To gain a more nuanced understanding of market trends, traders often employ multiple moving averages of varying lengths. For instance, using a short-term moving average like a 10-day SMA alongside a longer-term moving average such as a 50-day SMA can shed light on both immediate movements and long-term trends. A bullish signal is indicated when the short-term moving average crosses above the long-term average, while a bearish signal is suggested when it crosses below.
Moving averages are also effective in smoothing out short-term price volatility, making it easier to spot genuine trends. By averaging out the price over a set period, they reduce the impact of random fluctuations, offering a clearer view of the stock's trajectory.
Furthermore, moving averages can act as dynamic support and resistance levels. In an uptrend, the moving average often provides a support level where prices might rebound, while in a downtrend, it can serve as a resistance level, posing a challenge for prices to break through.
Crossovers of moving averages are particularly watched for as signals for entering or exiting trades. A bullish crossover, where a shorter-term moving average crosses above a longer-term average, suggests an upward momentum and a potential buying opportunity. On the other hand, a bearish crossover, where the shorter-term average falls below the longer-term one, indicates downward momentum and a possible selling point.
To enhance the reliability of their analysis, traders frequently combine moving averages with other technical indicators. For example, after identifying a moving average crossover, a trader might use an oscillator like the Relative Strength Index (RSI) to check whether the stock is overbought or oversold, thereby confirming the trend.
By effectively interpreting moving averages in these various ways, traders can make more informed decisions, whether they're considering entering a new position, exiting an existing one, or simply assessing the market's general sentiment.
Successful Moving Average Strategies
Moving averages have been central to many successful trading strategies, as evidenced by various case studies across different markets. These strategies showcase the effectiveness of moving averages in diverse market conditions and trading scenarios.
One prominent example is the use of the 'Golden Cross' and 'Death Cross' strategies in stock trading. The Golden Cross, a bullish signal, occurs when a short-term moving average like the 50-day SMA crosses above a long-term moving average such as the 200-day SMA. A case study of this might involve a large technology company's stock, where traders observed a significant uptrend following a Golden Cross, leading to substantial gains. On the other hand, the Death Cross, which signals bearish trends, happens when the short-term moving average crosses below the long-term average. An instance of this could be seen in a retail company's stock, where a Death Cross preceded a major downtrend, providing an opportunity for short sellers.
In the Forex market, traders often rely on Exponential Moving Averages (EMAs) due to their quick response to price changes. A common strategy involves using two EMAs, such as a 10-day and a 20-day EMA. A relevant case study might involve the EUR/USD currency pair, where a bullish signal was indicated by the 10-day EMA crossing above the 20-day EMA, resulting in profitable long positions. Conversely, a bearish signal emerged when the 10-day EMA crossed below the 20-day EMA, benefiting those in short positions.
Commodity trading also sees the application of moving average strategies, with traders often using Simple Moving Averages (SMAs) to navigate longer-term trends. For example, a trader might use a 100-day SMA to understand the overall trend and a shorter 20-day SMA for entry and exit signals. A case study in the crude oil market might demonstrate how a trader successfully bought when the price was above the 100-day SMA and sold when it fell below the 20-day SMA, capitalizing on the bullish market conditions.
In the highly volatile cryptocurrency market, combining moving averages with other indicators like the Relative Strength Index (RSI) has proven beneficial. Traders might use a 50-day SMA to identify the trend direction and RSI to spot overbought or oversold conditions. A case study involving a popular cryptocurrency, such as Bitcoin, could reveal instances where traders used a price above the 50-day SMA combined with an RSI reading below 30, indicating an oversold condition, to identify potential buy signals.
These case studies across various markets highlight the adaptability and effectiveness of moving average strategies. Whether in stocks, forex, commodities, or cryptocurrencies, moving averages, be they simple, weighted, or exponential, serve as potent tools for traders, especially when combined with other analytical techniques.
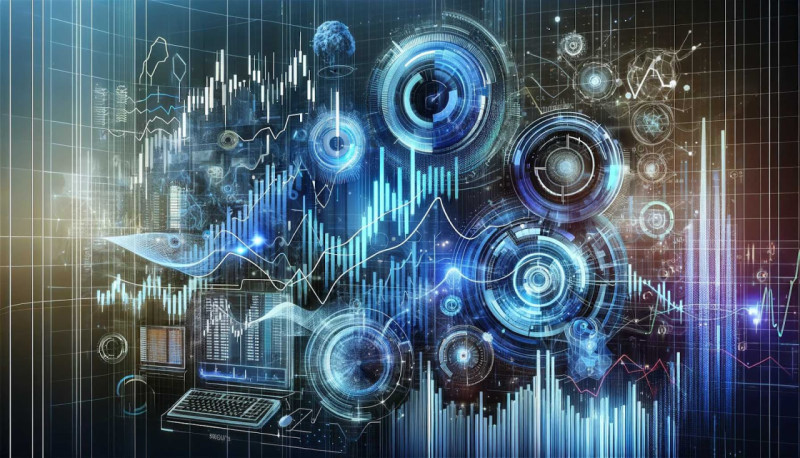
Moving Average Method in Forecasting
Application in Sales and Economic Forecasting
The moving average method is not only a valuable tool in financial trading but also plays a significant role in sales and economic forecasting. This method provides a robust framework for projecting future trends based on historical data, making it a popular choice among analysts and decision-makers in various industries.
In the realm of sales forecasting, the moving average method helps businesses predict future sales based on past sales data. This approach is particularly useful for identifying underlying sales trends free from seasonal fluctuations or irregular events. For instance, a retailer might use a 12-month moving average to forecast next year's sales, smoothing out the peaks and troughs associated with seasonal variations. By understanding the expected sales trend, businesses can make more informed decisions regarding inventory management, budget allocation, and strategic planning.
Economic forecasting also heavily relies on moving averages, especially for analyzing broad economic indicators such as GDP growth, unemployment rates, or inflation. Economists use moving averages to cleanse economic data of short-term volatility, allowing for a clearer understanding of long-term trends and cycles. For example, a moving average could be applied to quarterly GDP figures to gauge the underlying economic growth trend, stripping out the noise of short-term economic disruptions. This smoothed data provides a more accurate picture of the economy's health, assisting in policy formulation, investment decisions, and economic planning.
Moreover, the moving average method in economic forecasting can be instrumental in identifying turning points in economic cycles, such as recessions or recoveries. By observing when the moving average of an economic indicator changes direction, economists and policy-makers can get early signals of shifts in economic momentum.
Overall, the application of the moving average method in sales and economic forecasting is a testament to its versatility and effectiveness. By providing a clear view of trends and smoothing out irregularities, it enables businesses and economists to make predictions with greater confidence, ultimately leading to more strategic and well-informed decisions.
Comparative Analysis
The moving average method is one of several techniques used in forecasting, each with its unique strengths and applications. To understand the relative advantages and disadvantages of moving averages, it's helpful to compare this method with other popular forecasting techniques.
1. Moving Average vs. Exponential Smoothing:
- Moving averages provide a straightforward approach, calculating the average of data points over a set period. This method is excellent for smoothing out short-term fluctuations and highlighting longer-term trends.
- Exponential smoothing, on the other hand, assigns exponentially decreasing weights to older data points. It's more responsive to recent changes in the data, making it useful for short-term predictions in rapidly changing environments. However, this responsiveness can also make it more sensitive to recent fluctuations, potentially leading to overreaction in volatile conditions.
2. Moving Average vs. Linear Regression:
- Linear regression involves fitting a linear equation to historical data to predict future values. This method is powerful for identifying underlying trends and can be used for both short-term and long-term forecasting.
- Moving averages, while simpler, don't provide the predictive power of a linear regression model, as they don't account for other variables that might affect the data. However, their simplicity and ease of understanding make them more accessible for quick, general trend analysis.
3. Moving Average vs. ARIMA (Autoregressive Integrated Moving Average):
- ARIMA is a complex forecasting technique that combines autoregression, differencing (to make the data stationary), and a moving average. It's particularly effective for time series data where data points are interdependent.
- While the moving average method is part of ARIMA, the latter is more sophisticated and can model more complex patterns. However, ARIMA models require a deeper statistical understanding and are more complicated to set up and interpret.
4. Moving Average vs. Time Series Decomposition:
- Time series decomposition breaks down a time series into trend, seasonal, and random components. This method is useful for understanding different influences on the data and for making forecasts that account for seasonality.
- The moving average method primarily focuses on the trend component, smoothing out short-term fluctuations. It doesn't inherently separate out seasonality or randomness, which can be a limitation compared to decomposition methods.
In summary, while the moving average method is beneficial for its simplicity and effectiveness in highlighting trends, other forecasting methods offer advantages in terms of responsiveness, handling complex data structures, and accounting for various factors influencing the data. The choice of method depends on the specific requirements of the forecasting task, including the nature of the data, the forecasting horizon, and the level of detail and accuracy needed.
Challenges and Limitations in Forecasting
The moving average method in forecasting offers significant insights but also comes with inherent challenges and limitations that analysts and decision-makers need to be aware of.
A primary limitation is that the moving average is a lagging indicator. It relies on historical data, meaning its calculations are based on past trends. This characteristic can be a drawback in rapidly changing markets where recent developments or sudden shifts have not yet been fully reflected in the data. As a result, the moving average might not effectively predict future trends that deviate significantly from past patterns.
Another challenge is the potential over-simplification of data. The moving average method smooths out data fluctuations, which, while useful for highlighting general trends, can sometimes obscure important short-term variations. This smoothing effect can mask sudden market changes, anomalies, or seasonal effects, which could be crucial for certain types of analysis and decision-making.
The method's reliance on a defined time period for averaging is also a double-edged sword. Choosing the appropriate time frame is critical; too short a period might be too sensitive to temporary fluctuations, while too long a period could smooth out significant trends. This choice requires careful consideration and often a bit of trial and error, as the optimal period can vary depending on the nature of the data and the specific context of the forecast.
Moreover, moving averages inherently assume that past patterns and trends will continue into the future, which is not always the case. In markets or situations where the underlying dynamics are rapidly evolving, this assumption can lead to inaccurate forecasts. The method does not account for external factors or abrupt changes in the market environment, which can significantly impact future outcomes.
Lastly, while moving averages are useful for identifying trends, they do not provide insight into the reasons behind these trends. Understanding the causative factors is often crucial for comprehensive forecasting and strategic planning. Therefore, moving averages are usually more effective when used in conjunction with other analytical tools and methods that can offer additional insights into the underlying drivers of market changes.
In summary, while the moving average method is a valuable tool in forecasting, its effectiveness can be limited by its nature as a lagging indicator, potential for over-simplification, dependency on the chosen time period, assumption of future continuity of past trends, and lack of insight into causative factors. Users of this method should be aware of these limitations and consider complementing it with other analytical approaches for a more rounded and accurate forecasting process.
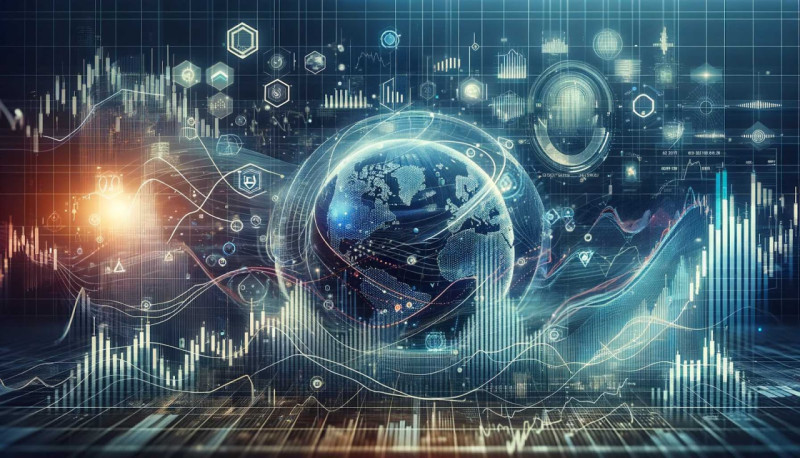
Calculating Moving Averages
Calculating moving averages is a fundamental aspect of their application in various analytical contexts. There are three primary types of moving averages - Simple Moving Average (SMA), Weighted Moving Average (WMA), and Exponential Moving Average (EMA), each with its unique calculation method.
Step-by-Step Calculation Process:
1. Simple Moving Average (SMA):
- Process: To calculate an SMA, sum up the closing prices of the asset for a specific number of periods (e.g., days, weeks, months) and then divide this total by the number of periods.
- Example: For a 10-day SMA, add the closing prices of the asset for the past 10 days and divide the sum by 10.
2. Weighted Moving Average (WMA):
- Process: The WMA assigns more weight to recent data points. The weights are typically linearly distributed. Multiply each price by its assigned weight, sum these values, and then divide by the sum of the weights.
- Example: In a 5-day WMA, the most recent price might be multiplied by 5, the next most recent by 4, and so on, down to the oldest price multiplied by 1. Sum these products and divide by the sum of the weights (1+2+3+4+5 = 15).
3. Exponential Moving Average (EMA):
- Process: EMA calculation involves more steps. Start with an SMA for the initial EMA value. Then, use the formulaEMA=(Closingprice−EMA(previousday))×multiplier+EMA(previousday), where the multiplier is 2÷(Numberofperiods+1).
- Example: For a 10-day EMA, the multiplier would be 2÷(10+1). Use this to adjust the EMA based on each day's closing price.
Comparative Analysis Table:
Type of Moving Average | Formula | Example Calculation | Pros | Cons
|
Simple Moving Average | SMA=(P1+P2+...+Pn)/n | SMA(10)=(Sumof10−dayclosingprices)/10 | Easy to calculate and understand | Can be slow to respond to new trends |
Weighted Moving Average | WMA=(Pn×n+Pn−1×(n−1)+...+P1×1)/(n+(n−1)+...+1) | WMA(5)=(P5×5+P4×4+...+P1×1)/15 | Gives more weight to recent data | More complex to calculate |
Exponential Moving Average | EMA=(Closingprice−EMA(previousday))×multiplier+EMA(previousday) | EMA(10)=(Today′sclosingprice−EMA(yesterday))×0.1818+EMA(yesterday) | Responsive to recent price changes | Requires an initial SMA for the first calculation |
This table and the described processes provide a clear overview of the different moving average calculations and their respective strengths and weaknesses. Each type has its specific use cases and can be selected based on the requirements of the analysis.
Advanced Techniques and Tools
Overview of Advanced Analytical Tools
In the financial analysis world, the use of moving averages has been greatly enhanced by advanced techniques and sophisticated software tools, catering to the nuanced needs of traders and analysts for precise, real-time, and comprehensive analyses.
1. Advanced Analytical Tools
- Algorithmic Trading Platforms - These platforms incorporate algorithmic capabilities, enabling traders to create and execute custom moving average strategies. Features often include backtesting, allowing traders to assess the effectiveness of their strategies against historical data.
- Charting Software - Advanced charting tools provide a variety of moving average types and settings. Traders can analyze multiple moving averages on different time frames and asset classes, with functionalities for detecting crossovers and other key signals.
- Technical Analysis Suites - These comprehensive tools blend moving average analysis with other technical indicators, like MACD, RSI, and Bollinger Bands, facilitating more nuanced market analysis.
2. Software for Calculating Moving Averages
- Financial Market Data Services - Platforms like Bloomberg and Reuters offer sophisticated analysis tools, including detailed moving average analytics. They provide real-time and historical data, essential for professional traders and analysts.
- Statistical Analysis Software - Software like MATLAB, R, and Python, especially with libraries like pandas and NumPy, are used for complex, customized analyses. These tools allow for the integration of moving averages into broader statistical models and can efficiently handle large datasets.
- Spreadsheet Software - Microsoft Excel and Google Sheets, while more basic, offer functionalities for calculating and plotting moving averages. They are ideal for quick analyses and widely used for educational purposes and by individual investors.
3. Emerging Technologies
- Artificial Intelligence and Machine Learning - These technologies are being increasingly applied to financial market analysis. They can optimize moving average parameters and identify complex patterns in market data.
- Cloud Computing and Big Data Analytics - The rise of cloud computing has made the analysis of vast datasets more accessible. Big data analytics, combined with cloud computing, enables the processing of massive amounts of market data for insights using moving averages and other statistical techniques.
The combination of these advanced tools and techniques significantly expands the capabilities of traders and analysts in employing moving averages, enhancing the efficiency and accuracy of their analyses and opening new possibilities for data-driven trading and investment strategies.
Integration of Moving Averages With Other Methods
Integrating moving averages with other analytical methods has become a staple in financial analysis, offering enhanced depth and effectiveness in understanding market dynamics. This multifaceted approach enables traders and analysts to validate their interpretations and make more informed decisions.
Combining moving averages with other technical indicators is a widely practiced strategy. For instance, using moving averages alongside the Relative Strength Index (RSI) allows traders to gauge both the trend direction and momentum of an asset. RSI can indicate overbought or oversold conditions, providing a context to the trend indicated by the moving average. Similarly, Bollinger Bands, which consist of a set of trend lines plotted two standard deviations away from a moving average, can be used in conjunction with moving averages to assess market volatility. When prices move outside the bands, it might signal a continuation or reversal of the trend, depending on the prevailing market conditions.
The Moving Average Convergence Divergence (MACD) is another tool often used with moving averages. MACD involves the calculation of two moving averages (a slow and a fast one) and their relationship, which can signal potential buy or sell opportunities. This integration is particularly useful in identifying trend reversals and momentum shifts.
In addition to technical indicators, moving averages are also combined with fundamental analysis. This approach is essential for traders who want to align technical indicators with broader economic indicators and company-specific financial metrics. For instance, a moving average might signal a bullish trend, but fundamental analysis, such as evaluating a company's earnings reports, market position, and economic conditions, can provide additional insights into whether the stock is a sound investment.
Another advanced integration involves statistical methods and machine learning. Techniques like regression analysis, time-series analysis, and even complex machine learning models can be used to analyze historical price data alongside moving averages. These methods can uncover more intricate patterns and relationships in the data, providing a more comprehensive understanding of market behavior.
Moreover, the use of moving averages in algorithmic trading strategies represents a sophisticated integration. Algorithms can be programmed to execute trades based on specific conditions involving moving averages, such as crossovers or the price relationship with moving averages. These automated systems can process vast amounts of data and execute trades at a speed and consistency challenging for human traders.
Integrating moving averages with these diverse analytical methods and tools offers a more holistic view of the markets. It helps in confirming trends, identifying potential investment opportunities, and managing risks more effectively. This comprehensive approach is crucial for navigating the complexities of financial markets and making well-informed trading decisions.
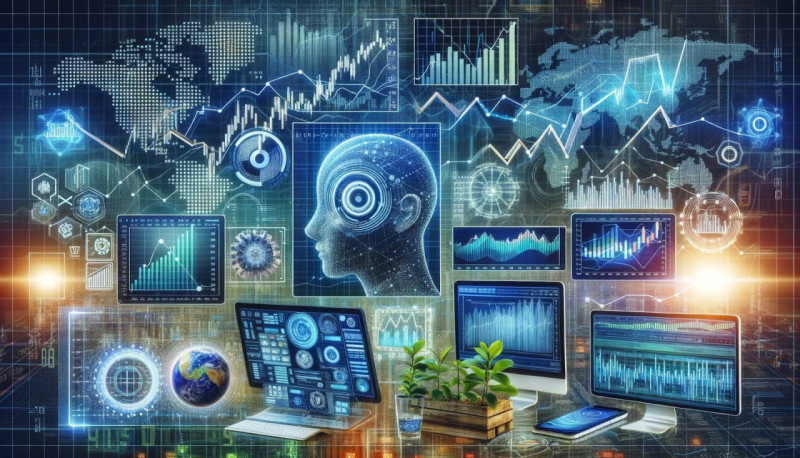
Future Trends and Developments
Emerging Trends in the Use of Moving Averages
The landscape of financial analysis is continually evolving, and with it, the application of moving averages is undergoing significant changes, influenced by advancements in technology and data analysis methods. These emerging trends are reshaping the way moving averages are utilized in market predictions and trading strategies.
One of the most notable trends is the integration of moving averages with machine learning and artificial intelligence (AI). This combination allows for more efficient analysis of vast market data volumes, far beyond the capabilities of traditional methods. Machine learning algorithms, armed with moving averages, can unearth complex market patterns and forecast future movements with enhanced accuracy. AI's ability to fine-tune the parameters of moving averages, such as their length and type, based on historical performance data, amplifies their predictive capacity.
The surge in big data analytics in finance is another critical trend influencing the use of moving averages. The availability of extensive, diverse datasets enables the application of moving averages across a wider range of variables. This approach is not just limited to traditional financial data but extends to social media sentiment, economic indicators, and even geopolitical events. Such comprehensive analysis offers a more holistic view of the factors influencing market conditions.
The rise of high-frequency trading (HFT) is also shaping the future use of moving averages. In HFT, algorithms execute a large number of orders at extremely high speeds. Moving averages are being adapted to operate in these fast-paced environments, providing rapid, real-time analysis that can be critical for the success of high-frequency trading strategies. These algorithms can make split-second decisions based on the slightest movements in moving averages, capitalizing on small price changes for substantial gains.
Furthermore, the increasing use of customized and adaptive moving averages is a trend to watch. Traders and analysts are experimenting with moving averages that can adapt to changing market conditions, adjusting their calculation periods and methodologies automatically in response to market volatility or other indicators. This adaptability makes moving averages more relevant and accurate in different market scenarios, enhancing their utility for traders.
In the future, we might also witness greater integration of moving averages with other emerging technologies such as blockchain and decentralized finance (DeFi) platforms. As financial markets continue to evolve, especially with the increasing digitalization and the introduction of new financial instruments, moving averages will likely be adapted to analyze and predict trends in these innovative spaces.
In summary, the future of moving averages in financial analysis looks dynamic and promising. With advancements in AI, big data analytics, high-frequency trading, and adaptive methodologies, moving averages are set to become even more sophisticated and integral to market analysis and trading strategy formulation.
Potential Future Applications and Research Areas
The future applications and research areas for moving averages are both diverse and expansive, stretching across various fields and integrating with advanced technological developments. As we look ahead, several key areas emerge where moving averages could play a pivotal role.
Enhanced market prediction models represent a significant area of potential application. Researchers are delving into integrating moving averages with advanced machine learning techniques like neural networks and deep learning algorithms. The aim is to create more accurate market prediction models that can incorporate a broader range of variables, including economic indicators, social media sentiment, and geopolitical events. These models promise to offer a more comprehensive understanding of market dynamics, potentially revolutionizing trading strategies.
Another promising research area is the application of moving averages in the burgeoning field of decentralized finance (DeFi) and blockchain technology. As these technologies mature, there's a growing opportunity to apply moving averages to analyze and predict trends in cryptocurrency markets and other digital financial instruments. This application could be particularly crucial in understanding the highly volatile and speculative nature of these markets.
The integration of moving averages with big data analytics is also an area ripe for further exploration. As businesses and financial institutions continue to collect vast amounts of data, the potential to apply moving averages to this data for trend analysis and forecasting is immense. This approach could extend beyond financial markets, impacting sectors like retail, healthcare, and manufacturing, where predictive analytics play a crucial role in strategic planning and decision-making.
Environmental science and climate change research are also emerging as fields where moving averages could have significant applications. Researchers could use moving averages to analyze environmental data trends, helping to predict changes in climate patterns, the spread of pollutants, or the effects of conservation policies. This application could be instrumental in shaping environmental strategies and policies.
Finally, the development of adaptive moving averages is a fascinating area for future research. These moving averages would automatically adjust their parameters based on changing market conditions, potentially offering more accurate and timely insights. This adaptability could be particularly beneficial in fast-paced and volatile markets, where traditional moving averages might lag behind rapid changes.
In summary, the future of moving averages looks to be marked by deeper integration with advanced technologies and broader applications across various sectors. From enhancing financial market models to aiding in climate change research, the potential of moving averages to provide valuable insights and predictions is vast and continues to grow with technological advancements.
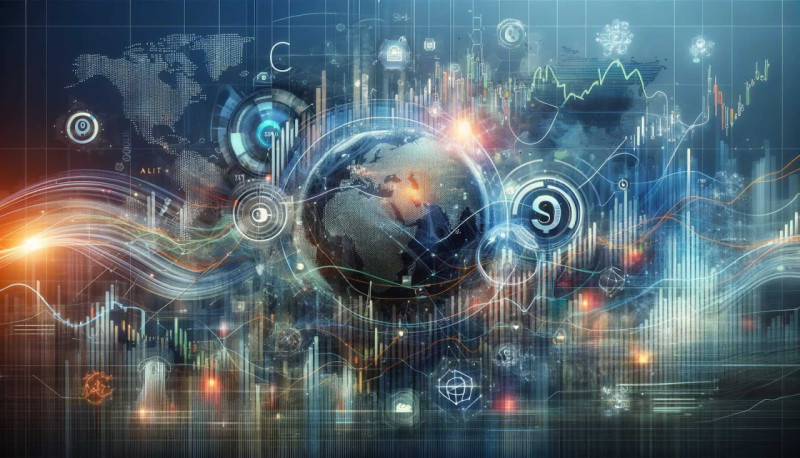
Conclusion
In this exploration of the moving average method, we have delved into various aspects that highlight its importance and versatility in financial analysis and beyond. From its fundamental concepts to its advanced applications, the moving average method stands out as a crucial tool in the arsenal of traders, analysts, and researchers.
We began by understanding the basic principles of moving averages, including their types - Simple Moving Average (SMA), Weighted Moving Average (WMA), and Exponential Moving Average (EMA). Each type serves a specific purpose, catering to different analytical needs. We then explored the application of moving averages in financial markets, particularly in stock market analysis, where they are instrumental in trend identification and setting up trading strategies.
The method's role in forecasting, especially in sales and economic forecasting, was also examined. Here, moving averages help in smoothing out data, allowing for clearer trend identification and prediction. However, we also acknowledged the challenges and limitations inherent in forecasting, such as the method's nature as a lagging indicator and potential oversimplification of data.
The technical aspects of calculating moving averages were covered, providing a deeper understanding of how these averages are derived and their comparative advantages and disadvantages. In the realm of advanced techniques and tools, we saw the integration of moving averages with sophisticated software and emerging technologies like AI and machine learning, opening up new possibilities for market analysis.
Looking to the future, we discussed the potential applications and research areas for moving averages, which span across market prediction models, decentralized finance, big data analytics, environmental science, and the development of adaptive moving averages.
In conclusion, the moving average method, with its simplicity, adaptability, and effectiveness, remains a fundamental component in the field of financial analysis. Its ability to filter out 'noise' and highlight underlying trends makes it invaluable for making informed decisions in various market conditions. As technology evolves, so too will the applications and sophistication of moving averages, further cementing their role as a versatile and essential tool in understanding and navigating the complex world of financial markets and beyond.
Recommended: